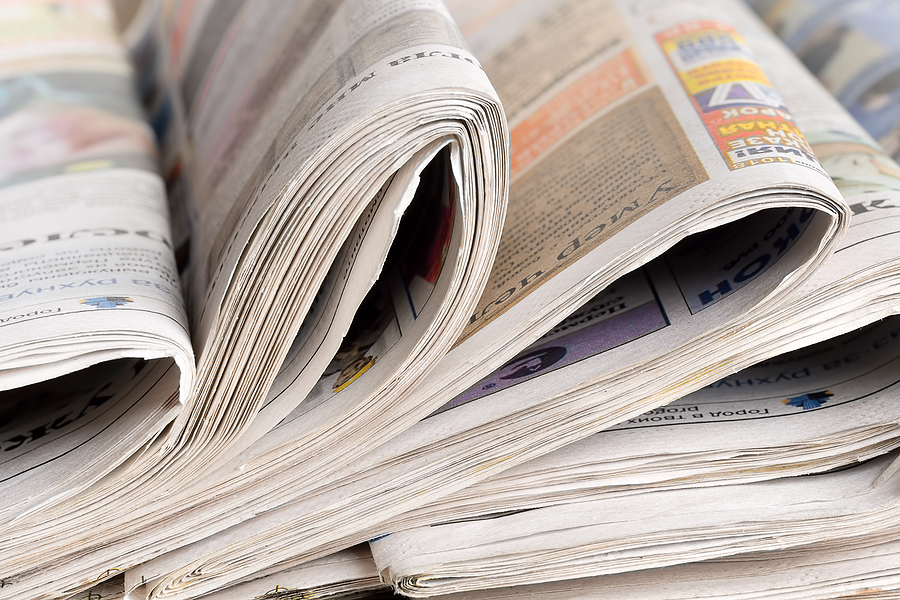
Can we automatically distinguish Russian from U.S. state-funded news?
Russian state-funded outlets play a key role in Russian disinformation campaigns abroad. A study in the International Journal of Communication tries to determine whether it is possible to automatically detect if a news item belongs to a U.S. outlet or a Russian state-funded outlet. Applying ‘machine learning’ technology, the researchers investigate the use of the topics covered and the complexity of the language used as features for the text classification task. The results are promising, and show that simple language characteristics can be particularly useful for automatically detecting the source of a news article on online news outlets or social media.
Take aways
- U.S. and Russian state-funded news outlets show considerable differences in their choice of topics and actors to focus on, as well as in their style of coverage. U.S. outlets have longer and simpler news items focused on domestic affairs, while Russian outlets use longer titles, more complex language, and promote anti-Western and pro-Russian narratives.
- Simple features, such as article length and language complexity, are particularly useful for text in languages for which limited automatic detection resources are available, such as Serbian. This means that automatic text classification does not always need a complex approach.
- The news frames captured in this study link to anti-Western attitudes, ethnic grievances, and revisionist nationalist themes that are more generally used by right-wing actors across Europe. This could mean that an automated ‘machine learning’ detector can be applied to social media to identify disinformation.
Study information
Where?
While the researchers were physically located in the Netherlands, the data they analyzed were from Serbian online outlets.
How?
The researchers scraped data from five online news outlets. Four trained coders coded the presence of topics (frames) which were expected to be present in articles from Russian-state funded outlets (pro-Russian sentiment, anti-West sentiment, etc.) in 1,000 articles. Based on these data, the researchers trained machine learning classifiers to identify the presence of these topics in the remaining 9,000 articles. After extracting linguistic features such as language complexity, average word length and named entities discussed in the text, the researchers combined this information to train a supervised machine learning classifier to automatically identify whether an outlet belongs to Russian or U.S. state-funded outlets. They then compared several combinations of these features to identify the best feature combination for the classifier.
Facts and findings
- While Russian state-funded outlets are often described as guises for promoting Russian political interest, much of the content shared by Russian outlets in Serbia resembled normal, informative news content, with at most 20% of articles containing anti-Western narratives.
- The researchers achieved a precision score of 75% in the country-source classification of news items, meaning that the model was both precise and robust in the majority of the classifications.
- Simple and easily obtained linguistic properties of text, such as article length, named entities used and language complexity, turned out to be very useful. Machine learning models based only on these features achieved a score of 73%, almost as high as the more complex approach.